The importance of data cannot be overstated—it fuels innovation, drives efficiencies, and serves as the backbone for most strategic decision-making. Data is the lifeblood of virtually every industry, and despite it not appearing on the balance sheet, it often stands as the most valuable asset an organisation possesses.
In this new era, enterprise test data management has a crucial role: ensuring that compliant, rich, and diverse datasets are readily available to teams whenever and wherever they are needed. Huw Price (co-founder of Curiosity Software) coined the phrase “Right Data, Right Place, Right Time” to this extent. This mantra is more relevant today than ever, driven by two primary factors:
Data Protection: With data’s immense value comes the critical responsibility to protect it. Data breaches can have devastating financial and irreparable reputational consequences.
Data Access: For various roles within an organisation—including developers, testers, data scientists, and business analysts—having access to realistic and varied data scenarios is essential. These scenarios are often absent in production, particularly when dealing with new functionalities or conducting complex analyses. Ensuring that all these teams have the data they need is crucial for their success and, by extension, the success of the enterprise.
See the future of test data
Learn how you can ensure "Right Data, Right Place, Right Time" across your organisation to boost productivity, enhance quality, and streamline your software delivery process.
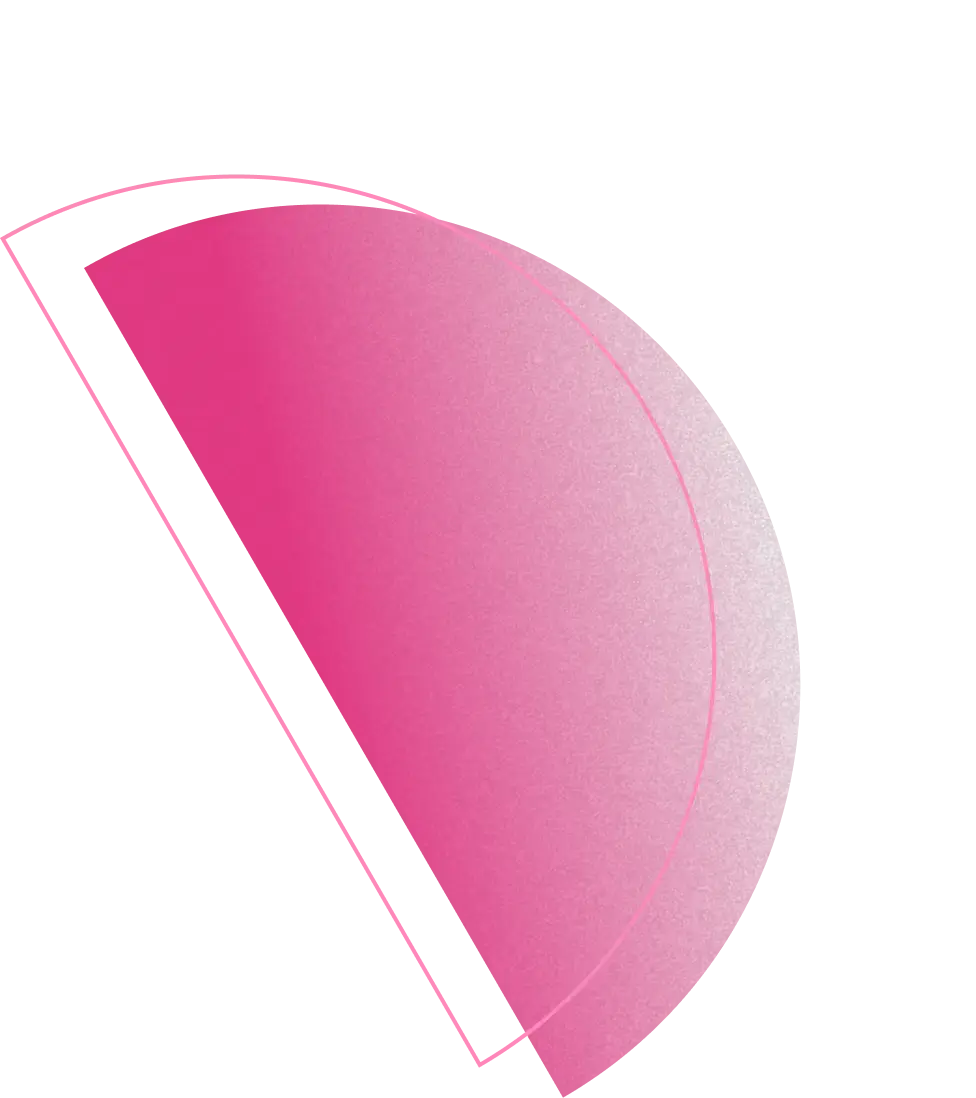
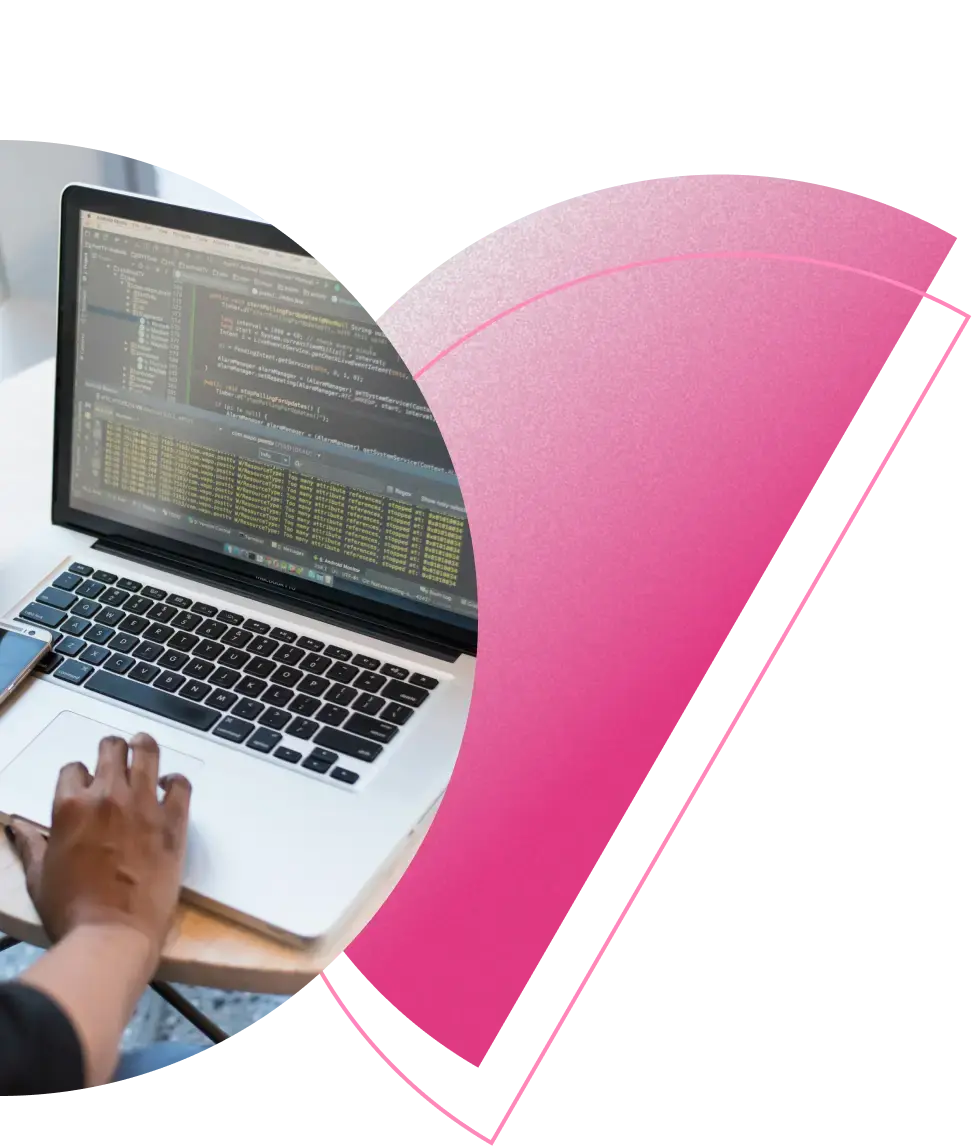
What’s changed?
While test data management is not a new discipline — discussions date back to the early 1980s— it has traditionally centered around relational databases. Historically, this involved masking or generating data within SQL-oriented databases and similar environments. While, relational databases are still vastly used, the data landscape has evolved dramatically. Organisations now deal with a vast array of data types, including NoSQL databases, messages, text files, XML, JSON, YAML, spreadsheets, the list goes on. The sheer number of databases and data formats in any organisation today underscores the complexity and breadth of the modern data ecosystem. Additionally, with the advent of multi-tier and microservice architectures, databases are often treated as ‘black box’ systems, with APIs serving as the primary gateway to backend data.
Data is also no longer the exclusive domain of developers and testers. Data scientists, business analysts, platform engineers and other professionals across the organisation require access to data for performing aggregations, conducting analyses, and deriving insights that inform critical business decisions. This broadened demand highlights the growing importance and need for enterprise test data across the entire organisation. Traditional test data tooling and processes struggle to meet these needs effectively, making a new approach necessary.
The inner & outer loops demand rich data
Recognising these challenges, Curiosity has pioneered a new approach to diagnose and address challenges within enterprise software delivery, dividing the process into inner and outer loops.
The Inner Loop is primarily focused on the developer’s day-to-day tasks, including writing code, debugging, and conducting unit tests. This loop is iterative and fast-paced, requiring immediate access to relevant data to ensure that code is tested in environments that closely mimic real-world scenarios.
The Outer Loop, on the other hand, encompasses the broader set of activities that support and surround the inner loop. This includes requirements gathering, integration testing, environment provisioning, and, critically, data management. The outer loop operates on a longer timeline but is equally dependent on having rich data available to ensure that the software delivery process is efficient and effective.
Both loops are heavily reliant on high-quality, compliant data to function effectively. When adequate data isn't available, both quality and productivity suffer, leading to bottlenecks and suboptimal outcomes. The quality of testing is directly tied to the quality of the data; you can only test as thoroughly as the data allows. The challenge lies in managing and provisioning this data across the inner (technical) and outer (non-technical) loops to avoid bottlenecks and ensure seamless integration throughout delivery.
The problem is compounded when production data is used inappropriately, a practice that is unsustainable due to privacy concerns and data integrity issues. As applications become increasingly data-centric, the need for rich data has never been more critical. The proliferation of diverse data types amplifies the complexity of managing test data.
Requirements of a modern enterprise test data platform
The new world of enterprise test data must address these challenges head-on to support the dynamic needs of today’s organisations. Here are the key requirements for a modern enterprise test data platform and the future of test data.
#1 – Navigating data complexity across systems
The modern data landscape is far more complex, with organisations now relying on a multitude of interconnected systems. These systems generate and manage diverse data types and formats, ranging from NoSQL databases and message queues to cloud storage and APIs. This interconnected web of data sources introduces significant complexity in test data management, as it requires not only the generation or masking of data from individual systems but also the orchestration of data across these varied platforms to create comprehensive and accurate test scenarios.
Enterprise Test Data (ETD) must now be capable of seamlessly connecting across these diverse sources to support complex testing needs. The challenge lies in ensuring that data can be accessed, transformed, and utilised effectively across all systems involved in the software delivery process. As applications increasingly depend on distributed data ecosystems, managing this complexity is crucial for maintaining data quality, compliance, and availability.
#2 – Adapting to an API first application landscape
The shift from thick client and monolithic architecture applications to an API-led approach has been driven largely by the adoption of SaaS applications within enterprises. In this API-first environment, interactions typically occur solely through APIs, with little to no direct access or concept of the underlying backend databases.
This shift necessitates a departure from traditional test data management, which relies heavily on relational databases. Instead, modern test data platforms must ensure interoperability with APIs to provide compliant data efficiently. APIs often match the speed of direct database calls with minimal overhead, rendering direct database access unnecessary even when technically feasible. Consequently, a modern test data management platform must excel in generating and handling various message formats (such as XML and JSON), retrieving data through APIs, and chaining API calls into sophisticated sequences. Effective message handling and generation are now critical components of contemporary test data management.
A notable example of this shift is the financial industry’s transition from the proprietary SWIFT payment messaging system to ISO 20022, an open XML-based format. This change underscores how adopting standardised API communication protocols can streamline and enhance financial transactions.
#3 – Enhancing data coverage with visual modelling
Ensuring comprehensive data coverage requires more than just relying on repetitive production data. Visual modelling offers a dynamic approach to capturing the full breadth of necessary data scenarios. By using intuitive and visual diagrams, users can graphically represent and embed business rules and logic directly into the data creation process. This method allows teams to define precise data scenarios, ensuring all potential variations are considered. Once defined, these models can generate data across various platforms—whether JSON, relational databases, NoSQL, or other formats—abstracting away from the underlying data technologies and maintaining consistency across environments.
Visual modelling can also integrate with AI to further enhance the quality and relevance of generated test data. AI-powered co-pilots can assist in building equivalence classes and accelerating model creation, allowing teams to quickly generate comprehensive data scenarios from textual requirements. This approach not only ensures richer and more accurate test coverage but also enables organisations to efficiently meet the demands of software delivery.
#4 – Platform extensibility: Seamless data access across any application
In modern software delivery, different teams—from developers and testers to data scientists and business analysts—require access to a variety of data types and formats tailored to specific systems and use cases. These diverse data needs highlight the importance of a test data management platform that is both flexible and extensible, capable of adapting to the unique requirements of each team and system.
Given the vast array of applications within an enterprise, no single platform can provide out-of-the-box support for every data source or format. Therefore, an extensible platform is essential, allowing seamless integration with various data sources and accommodating specific configurations across the organisation. This flexibility ensures that all teams can access the high-quality, compliant data they need, when they need it, supporting efficient and effective software delivery.
#5 – AI accelerated test data
AI is redefining the possibilities within software, particularly in the traditionally stagnant field of test data management, which has seen little innovation beyond the addition of new data sources. Historically, organisations have struggled with a lack of structured documentation, making it difficult to fully understand and access the data they need for specific tasks. This limitation has hindered the ability to apply effective test data techniques, leaving teams without the rich, well-understood datasets necessary to perform their work efficiently. Large language models (LLMs) are now disrupting this space by offering more intuitive ways to interact with data, to provide a more comprehensive and accessible view of an organisation’s data landscape.
The emergence of AI co-pilots and virtual data engineer agents will further empower stakeholders to interact with their data, gaining instant insights and generating data on-the-fly. This advancement not only streamlines data management but also democratises access to complex data tasks, enabling anyone within the organisation to efficiently retrieve and manipulate data without needing deep technical knowledge.
#6 – Natural language interface for test data
AI-driven natural language interfaces, similar to the popular ChatGPT are disrupting complex approaches to simple tasks performed via chatting to an AI. These interfaces will enable users to interact directly with their data, allowing them to ask questions, gain insights, and receive summaries with ease. By leveraging a well-configured data dictionary that maps the existing data landscape, these AI tools will make data querying and management more intuitive and accessible, empowering users across the organisation, regardless of their technical expertise.
Beyond knowledge retrieval, these chat interfaces will integrate with specialised agents capable of executing complex test data activities based on user guidance. Users will be able to instruct these agents to find, create, and manipulate data within backend sources, all through natural language commands. Visual models will play a crucial role in this process, allowing users to understand what an AI has done to build trust, and express comprehensive scenarios to ensure adequate data coverage.
The Curiosity Enterprise Test Data® Platform
The evolution of test data management underscores the increasing significance of data as the lifeblood of modern enterprises. Traditionally centred on relational databases, the focus has now broadened to encompass a diverse array of data types, reflecting the complexity and breadth of today’s data landscape. As organisations increasingly depend on data to build and test modern applications, the demand for high-quality, compliant test data has reached an unprecedented level of importance.
To address these challenges, Curiosity has pioneered the world’s first Outer Loop Centric Platform—a holistic approach that supports the entire software delivery process. This platform seamlessly integrates the fast-paced inner loop of development with the broader outer loop of requirements, testing, and test data—areas that are often the most significant challenges and barriers to productivity and quality.
Curiosity’s enterprise test data capabilities are specifically designed for this new era, ensuring that data is always available, compliant, and of the highest quality. This robust framework not only addresses the complexities of today’s data environment but also positions organisations to excel in the data-driven future.
As the landscape of test data management continues to evolve, advancements in AI, visual modelling, and natural language interfaces will be crucial in making data more accessible and valuable across the enterprise. Curiosity’s Enterprise Test Data® platform remains at the forefront of this evolution, providing a solution that is fully equipped to meet the data demands of tomorrow.
Full data coverage, on demand
Speak to a Curiosity expert to learn how we can help you deliver rich synthetic data, available on demand across your entire enterprise, with the Outer Loop Platform.
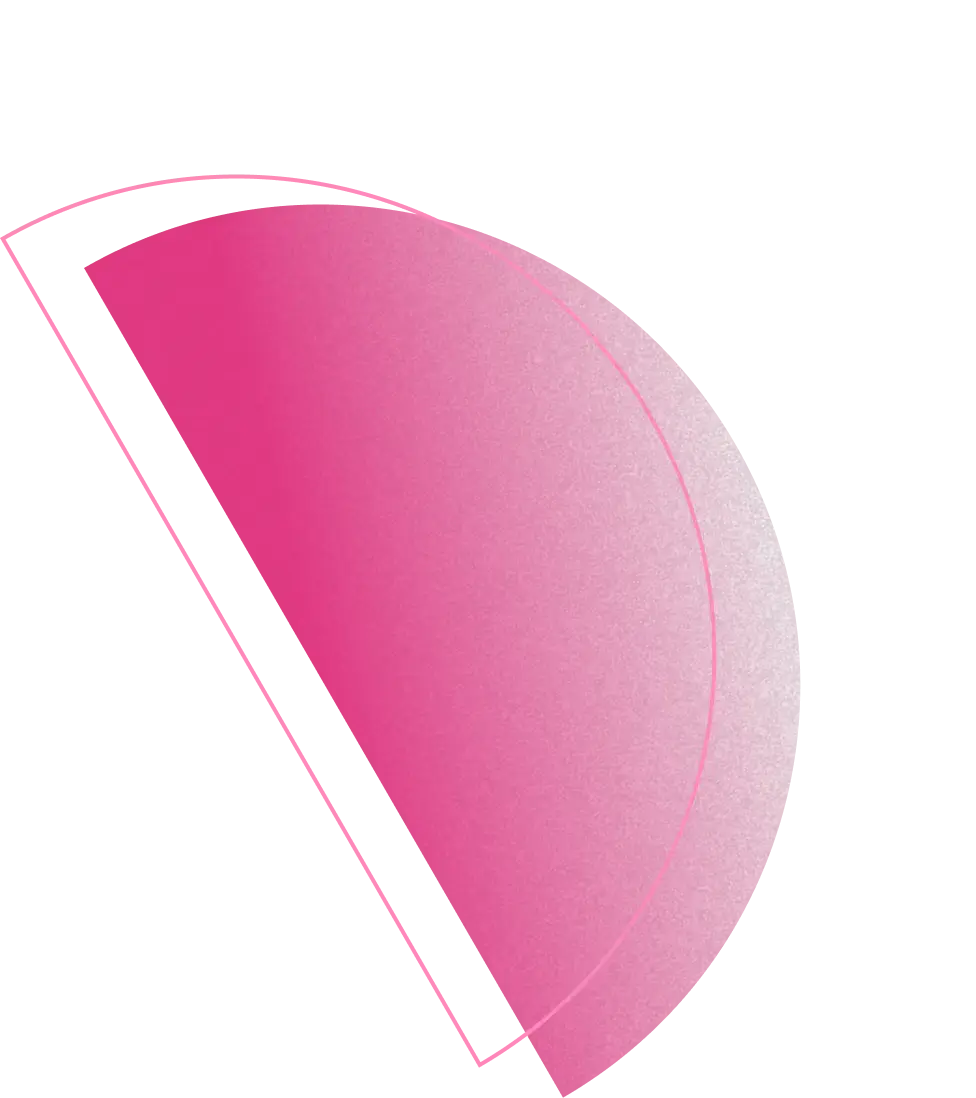
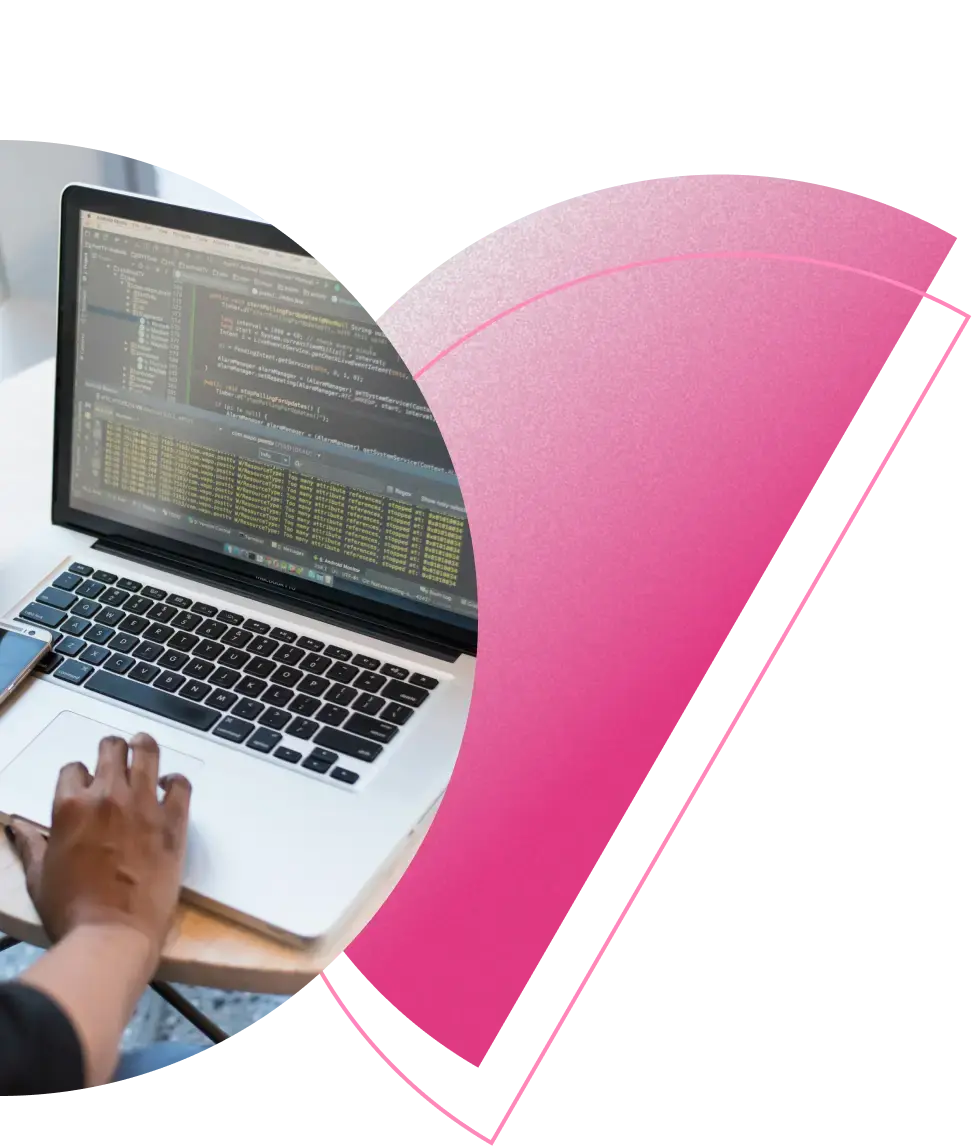